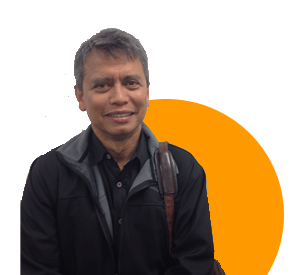
My son and I are both similar and different. I am human and so he is. He is male and so am I. He’s got brown eyes and black hair and so do I. There are the usual physical look alike traits and mannerisms and yet while similar we are also different. I am old and he is young. He likes to listen to dub step and I like my classical music. And because of our age difference, he looks at the world from a very different perspective. So we are similar in some ways and different in other ways.
A segmentation model is built on these principles. The model measures similarities and differences of customers so that marketers can better communicate with them. By observing the differences between two customers, they can customise direct mail outs that are more relevant, i.e., personal. Instead of sending one generic message, marketers go below the line and go to customers with personalised offers.
To make this happen, marketers often use a segmentation model. Its purpose is to group together customers with similar characteristics. The same segmenting of customers also highlight the differences among them.
To group together similar objects requires a criteria that defines “similarity”. Take the basic segmentation model in marketing known as the RFM model. The model uses a cell based approach. RFM stands for recency, frequency and monetary value. These three attributes or criteria determine whether a customer (an object) is similar to another customer. If Customer A has a recency of 5 days, a frequency of 4 visits in a month and spends $100 per visit on average then someone with a recency of 4 days, a frequency of 6 visits in a month and spends $95 per visit on average will be similar to Customer A and they get segmented in the same group. Customer C may have similar attributes with Customer A on recency and frequency but has a significantly different monetary value ($200 per visit on average) and will end up being grouped with another set of customers having similar characteristics. It is all about trying to be similar in most if not all of the attributes.
In real life, a customer can have heaps of attributes depending on how good the data is about that customer. Marketers’ utopia is a world where very detailed information about a customer can be readily access. But we need to trim the list and identify those set of characteristics which will lead to the “right” segmentation model.
So going from three attributes to a thousand attributes requires some type of methodology that can group similar objects together based on those attributes on a consistent basis. An iteration method known as the k-means algorithm is one such tool. Using a mathematical formula, an n-dimension space is mapped out and objects that are ‘near’ to each other are considered similar. It starts with a predefined “k” and then starts grouping objects according to a set of criteria until all objects become one group. The process is mapped out and an optimal “k” determines the best set of clusters.
Most segmenting or clustering techniques can be classified into two types – disjoint or hierarchical. The disjoint method ensures that each object belongs to one and only one segment or cluster.
In a hierarchical method, objects can belong to more than one cluster or segment. In the case of the k-means algorithm, objects are mapped in a Dendogram showing the different levels of similarity and its corresponding groupings. On the one end of the spectrum are individual objects each representing a unique cluster. The other end of the spectrum shows a single group representing all objects. The process of going from many to one segment is achieved using the k-means algorithm.
How do we know we have a good segmentation model? If the model is able to differentiate between objects (e.g., customers) based on some criteria we know to be true, then the model is working. With the k-means algorithm, it is about identifying the right “k” that results in the best set of clusters or segments.
And like other analytical tools, a segmentation model has a shelf life. Sooner or later the segments fail to capture the distinct similarities and differences of the groups. Assessing how well the model performs is to look at each object distance to the mean of their cluster in repeated sampling. If the distances change substantially across repeats, then it is time to have a rebuild of the model.
Roberto.