The Analytics and Data Mining tools space is growing prolifically, new startups keep popping up and raising various masses of cash to build their product out – many disappearing as the result of an early stage sale or running out of said cash too. There are loads of taster articles to give you an idea of the scope like this one 15 Big Data and Analytics Companies to Watch for instance. Industry commentators love this space too, here on KDNuggests is a comparison of the Gartner Magic Quadrants for 2014 and 2015 in Predictive Analytics tools, Forbes posted an article listing the Top 100 Analytics Startups in 2015 part way through last year (with a download to Excel option). These ratings and list inclusions are heavily weighted on how much ca$h each company has raised – which in itself implies a vast customer base and feature rich product (benefit of the doubt given to tech investors on my part here).
Most of the tools listed in these articles offer a commercial product, some, however, have an Open Source with Premium path offering as well. I started thinking about this back in August at the RapidMiner 2015 conference set in a beautiful Slovenian castle – yes, it was amazing. I was talking to Data Scientists, Medical Researchers, GP’s and Ph.D. Candidates who all started (and many continue) their journeys into Data Mining or Predictive Analytics by downloading the Open Source version of RapidMiner.
This discovery coupled with living in the land of “R” where students are emerging armed with newfound statistical modelling and data mining know how all honed using “R” has created demand for Open Source and R derivative products. To get your started here is a short list of tools you can download and use, their Open Source license Attribution and a link to find them, as much as I don’t always trust Wikipedia it has proven a good source of non-techo descriptive information on each product below. A short list, varied in maturity and finesse with only Rapidminer having morphed into what is now a large commercial player.
RapidMiner is a software platform developed by the company of the same name that provides an integrated environment for machine learning, data mining, text mining, predictive analytics and business analytics.
- License attributions: Basic (Open Source), Community (Open Source) and Professional (commercial)
- Open Source license attribution: AGPL-3.0
- Download link https://rapidminer.com/products/studio/
- Find out more https://rapidminer.com/products/comparison/
Apache Mahout is a project of the Apache Software Foundation to produce free implementations of distributed or otherwise scalable machine learning algorithms focused primarily in the areas of collaborative filtering, clustering and classification.
- License attributions: Open Source
- Open Source license attribution: Apache License
- Download link https://mahout.apache.org/general/downloads.html
- Find out more http://mahout.apache.org/general/faq.html#whatis
Orange is a comprehensive, component-based software suite for machine learning and data mining, developed at Bioinformatics Laboratory, Faculty of Computer and Information Science, University of Ljubljana, Slovenia, together with open source community.
- License attributions: Open Source
- Open Source license attribution: GPL 3.0
- Download link http://orange.biolab.si/download/
- Find out more http://blog.biolab.si
Waikato Environment for Knowledge Analysis (Weka) is a popular suite of machine learning software written in Java, developed at the University of Waikato, New Zealand. It is free software licensed under the GNU General Public License.
- License attributions: Open Source
- Open Source license attribution: GPL 3.0
- Download link http://www.cs.waikato.ac.nz/ml/weka/downloading.html
- Find out more http://www.cs.waikato.ac.nz/ml/weka/ or their cool MOOC site https://weka.waikato.ac.nz/explorer
DataMelt (or, in short, DMelt) a computation and visualization environment, is an interactive framework for scientific computation, data analysis and data visualization designed for scientists, engineers and students.
- License attributions: Open Source
- Open Source license attribution: GPL 3.0
- Download link http://jwork.org/dmelt/index.php?id=install
- Find out more http://jwork.org/dmelt/index.php?id=about
Knowledge Extraction based on Evolutionary Learning (KEEL) provides a simple GUI based on data flow to design experiments with different datasets and computational intelligence algorithms (paying special attention to evolutionary algorithms) in order to assess the behaviour of the algorithms.
- License attributions: Open Source
- Open Source license attribution: GPL 3.0
- Download link http://sci2s.ugr.es/keel/download.php
- Find out more http://sci2s.ugr.es/keel/description.php
SPMF is an open-source data mining mining library written in Java, specialized in pattern mining.
- License attributions: Open Source
- Open Source license attribution: GPL 3.0
- Download link http://www.philippe-fournier-viger.com/spmf/index.php?link=download.php
- Find out more http://data-mining.philippe-fournier-viger.com/tag/spmf/
Rattle GUI is a free and open source software package providing a graphical user interface (GUI) for data mining using the R statistical programming language.
- License attributions: Open Source
- Open Source license attribution: GPL 2.0
- Download link http://rattle.togaware.com/rattle-download.html
- Find out more http://rattle.togaware.com/
Hope you have found something useful here.
Now Just Because It’s Fun!
If you want to make a quick easy visualization of your dataset try Raw – it’s so easy I can do it! This took me 2 minutes. I downloaded the CSV of ACC Claims from data.govt.nz and pasted in the injury claim totals by region 2014 rows into Raw, filtered on Females and voila! It’s crude because it took me 2 minutes, imagine if I had spent 5, it could be gorgeous.
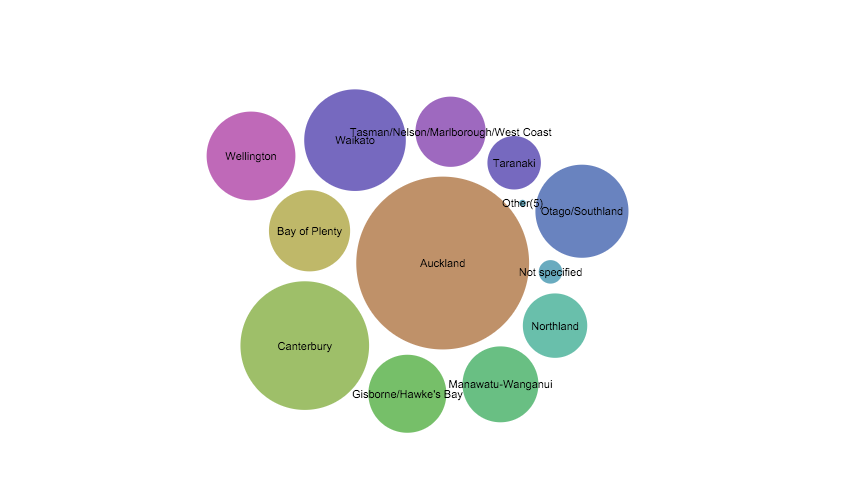
Source: OptimalBI
Enjoy. Vic.